โมเดล Gemini สร้างขึ้นมาใหม่ทั้งหมดให้ทำงานได้หลายรูปแบบ ซึ่งจะช่วยให้คุณทำงานด้านการประมวลผลรูปภาพและคอมพิวเตอร์วิทัศน์ได้หลากหลาย รวมถึงแต่ไม่จำกัดเพียงการสร้างคำบรรยายรูปภาพ การจัดประเภท และการตอบคำถามเกี่ยวกับภาพโดยไม่ต้องฝึกโมเดล ML เฉพาะทาง
ส่งรูปภาพไปยัง Gemini
คุณสามารถระบุรูปภาพเป็นอินพุตให้กับ Gemini ได้ 2 วิธี ดังนี้
- การส่งข้อมูลรูปภาพในบรรทัด: เหมาะสำหรับไฟล์ขนาดเล็ก (คำขอทั้งหมด มีขนาดน้อยกว่า 20 MB รวมถึงพรอมต์)
- การอัปโหลดรูปภาพโดยใช้ File API: แนะนำสำหรับไฟล์ขนาดใหญ่หรือสำหรับ การนำรูปภาพไปใช้ซ้ำในคำขอหลายรายการ
การส่งข้อมูลรูปภาพในบรรทัด
คุณส่งข้อมูลรูปภาพในหน้าได้ในคำขอไปยัง generateContent
คุณระบุข้อมูลรูปภาพเป็นสตริงที่เข้ารหัส Base64
หรือโดยการอ่านไฟล์ในเครื่องโดยตรง (ขึ้นอยู่กับภาษา) ได้
ตัวอย่างต่อไปนี้แสดงวิธีอ่านรูปภาพจากไฟล์ในเครื่องและส่งไปยัง API generateContent
เพื่อประมวลผล
Python
from google.genai import types
with open('path/to/small-sample.jpg', 'rb') as f:
image_bytes = f.read()
response = client.models.generate_content(
model='gemini-2.5-flash',
contents=[
types.Part.from_bytes(
data=image_bytes,
mime_type='image/jpeg',
),
'Caption this image.'
]
)
print(response.text)
JavaScript
import { GoogleGenAI } from "@google/genai";
import * as fs from "node:fs";
const ai = new GoogleGenAI({});
const base64ImageFile = fs.readFileSync("path/to/small-sample.jpg", {
encoding: "base64",
});
const contents = [
{
inlineData: {
mimeType: "image/jpeg",
data: base64ImageFile,
},
},
{ text: "Caption this image." },
];
const response = await ai.models.generateContent({
model: "gemini-2.5-flash",
contents: contents,
});
console.log(response.text);
Go
bytes, _ := os.ReadFile("path/to/small-sample.jpg")
parts := []*genai.Part{
genai.NewPartFromBytes(bytes, "image/jpeg"),
genai.NewPartFromText("Caption this image."),
}
contents := []*genai.Content{
genai.NewContentFromParts(parts, genai.RoleUser),
}
result, _ := client.Models.GenerateContent(
ctx,
"gemini-2.5-flash",
contents,
nil,
)
fmt.Println(result.Text())
REST
IMG_PATH="/path/to/your/image1.jpg"
if [[ "$(base64 --version 2>&1)" = *"FreeBSD"* ]]; then
B64FLAGS="--input"
else
B64FLAGS="-w0"
fi
curl "https://generativelanguage.googleapis.com/v1beta/models/gemini-2.5-flash:generateContent" \
-H "x-goog-api-key: $GEMINI_API_KEY" \
-H 'Content-Type: application/json' \
-X POST \
-d '{
"contents": [{
"parts":[
{
"inline_data": {
"mime_type":"image/jpeg",
"data": "'"$(base64 $B64FLAGS $IMG_PATH)"'"
}
},
{"text": "Caption this image."},
]
}]
}' 2> /dev/null
นอกจากนี้ คุณยังดึงข้อมูลรูปภาพจาก URL แปลงเป็นไบต์ และส่งไปยัง generateContent
ได้ตามตัวอย่างต่อไปนี้
Python
from google import genai
from google.genai import types
import requests
image_path = "https://goo.gle/instrument-img"
image_bytes = requests.get(image_path).content
image = types.Part.from_bytes(
data=image_bytes, mime_type="image/jpeg"
)
client = genai.Client()
response = client.models.generate_content(
model="gemini-2.5-flash",
contents=["What is this image?", image],
)
print(response.text)
JavaScript
import { GoogleGenAI } from "@google/genai";
async function main() {
const ai = new GoogleGenAI({});
const imageUrl = "https://goo.gle/instrument-img";
const response = await fetch(imageUrl);
const imageArrayBuffer = await response.arrayBuffer();
const base64ImageData = Buffer.from(imageArrayBuffer).toString('base64');
const result = await ai.models.generateContent({
model: "gemini-2.5-flash",
contents: [
{
inlineData: {
mimeType: 'image/jpeg',
data: base64ImageData,
},
},
{ text: "Caption this image." }
],
});
console.log(result.text);
}
main();
Go
package main
import (
"context"
"fmt"
"os"
"io"
"net/http"
"google.golang.org/genai"
)
func main() {
ctx := context.Background()
client, err := genai.NewClient(ctx, nil)
if err != nil {
log.Fatal(err)
}
// Download the image.
imageResp, _ := http.Get("https://goo.gle/instrument-img")
imageBytes, _ := io.ReadAll(imageResp.Body)
parts := []*genai.Part{
genai.NewPartFromBytes(imageBytes, "image/jpeg"),
genai.NewPartFromText("Caption this image."),
}
contents := []*genai.Content{
genai.NewContentFromParts(parts, genai.RoleUser),
}
result, _ := client.Models.GenerateContent(
ctx,
"gemini-2.5-flash",
contents,
nil,
)
fmt.Println(result.Text())
}
REST
IMG_URL="https://goo.gle/instrument-img"
MIME_TYPE=$(curl -sIL "$IMG_URL" | grep -i '^content-type:' | awk -F ': ' '{print $2}' | sed 's/\r$//' | head -n 1)
if [[ -z "$MIME_TYPE" || ! "$MIME_TYPE" == image/* ]]; then
MIME_TYPE="image/jpeg"
fi
# Check for macOS
if [[ "$(uname)" == "Darwin" ]]; then
IMAGE_B64=$(curl -sL "$IMG_URL" | base64 -b 0)
elif [[ "$(base64 --version 2>&1)" = *"FreeBSD"* ]]; then
IMAGE_B64=$(curl -sL "$IMG_URL" | base64)
else
IMAGE_B64=$(curl -sL "$IMG_URL" | base64 -w0)
fi
curl "https://generativelanguage.googleapis.com/v1beta/models/gemini-2.5-flash:generateContent" \
-H "x-goog-api-key: $GEMINI_API_KEY" \
-H 'Content-Type: application/json' \
-X POST \
-d '{
"contents": [{
"parts":[
{
"inline_data": {
"mime_type":"'"$MIME_TYPE"'",
"data": "'"$IMAGE_B64"'"
}
},
{"text": "Caption this image."}
]
}]
}' 2> /dev/null
การอัปโหลดรูปภาพโดยใช้ File API
หากต้องการใช้ไฟล์ขนาดใหญ่หรือใช้ไฟล์รูปภาพเดียวกันซ้ำๆ ให้ใช้ Files API โค้ดต่อไปนี้จะอัปโหลดไฟล์รูปภาพ แล้วใช้ไฟล์ใน
การเรียกใช้ generateContent
ดูข้อมูลเพิ่มเติมและตัวอย่างได้ที่คู่มือ Files API
Python
from google import genai
client = genai.Client()
my_file = client.files.upload(file="path/to/sample.jpg")
response = client.models.generate_content(
model="gemini-2.5-flash",
contents=[my_file, "Caption this image."],
)
print(response.text)
JavaScript
import {
GoogleGenAI,
createUserContent,
createPartFromUri,
} from "@google/genai";
const ai = new GoogleGenAI({});
async function main() {
const myfile = await ai.files.upload({
file: "path/to/sample.jpg",
config: { mimeType: "image/jpeg" },
});
const response = await ai.models.generateContent({
model: "gemini-2.5-flash",
contents: createUserContent([
createPartFromUri(myfile.uri, myfile.mimeType),
"Caption this image.",
]),
});
console.log(response.text);
}
await main();
Go
package main
import (
"context"
"fmt"
"os"
"google.golang.org/genai"
)
func main() {
ctx := context.Background()
client, err := genai.NewClient(ctx, nil)
if err != nil {
log.Fatal(err)
}
uploadedFile, _ := client.Files.UploadFromPath(ctx, "path/to/sample.jpg", nil)
parts := []*genai.Part{
genai.NewPartFromText("Caption this image."),
genai.NewPartFromURI(uploadedFile.URI, uploadedFile.MIMEType),
}
contents := []*genai.Content{
genai.NewContentFromParts(parts, genai.RoleUser),
}
result, _ := client.Models.GenerateContent(
ctx,
"gemini-2.5-flash",
contents,
nil,
)
fmt.Println(result.Text())
}
REST
IMAGE_PATH="path/to/sample.jpg"
MIME_TYPE=$(file -b --mime-type "${IMAGE_PATH}")
NUM_BYTES=$(wc -c < "${IMAGE_PATH}")
DISPLAY_NAME=IMAGE
tmp_header_file=upload-header.tmp
# Initial resumable request defining metadata.
# The upload url is in the response headers dump them to a file.
curl "https://generativelanguage.googleapis.com/upload/v1beta/files" \
-H "x-goog-api-key: $GEMINI_API_KEY" \
-D upload-header.tmp \
-H "X-Goog-Upload-Protocol: resumable" \
-H "X-Goog-Upload-Command: start" \
-H "X-Goog-Upload-Header-Content-Length: ${NUM_BYTES}" \
-H "X-Goog-Upload-Header-Content-Type: ${MIME_TYPE}" \
-H "Content-Type: application/json" \
-d "{'file': {'display_name': '${DISPLAY_NAME}'}}" 2> /dev/null
upload_url=$(grep -i "x-goog-upload-url: " "${tmp_header_file}" | cut -d" " -f2 | tr -d "\r")
rm "${tmp_header_file}"
# Upload the actual bytes.
curl "${upload_url}" \
-H "x-goog-api-key: $GEMINI_API_KEY" \
-H "Content-Length: ${NUM_BYTES}" \
-H "X-Goog-Upload-Offset: 0" \
-H "X-Goog-Upload-Command: upload, finalize" \
--data-binary "@${IMAGE_PATH}" 2> /dev/null > file_info.json
file_uri=$(jq -r ".file.uri" file_info.json)
echo file_uri=$file_uri
# Now generate content using that file
curl "https://generativelanguage.googleapis.com/v1beta/models/gemini-2.5-flash:generateContent" \
-H "x-goog-api-key: $GEMINI_API_KEY" \
-H 'Content-Type: application/json' \
-X POST \
-d '{
"contents": [{
"parts":[
{"file_data":{"mime_type": "'"${MIME_TYPE}"'", "file_uri": "'"${file_uri}"'"}},
{"text": "Caption this image."}]
}]
}' 2> /dev/null > response.json
cat response.json
echo
jq ".candidates[].content.parts[].text" response.json
การป้อนพรอมต์ด้วยรูปภาพหลายรูป
คุณระบุรูปภาพหลายรูปในพรอมต์เดียวได้โดยรวมออบเจ็กต์รูปภาพหลายรูป
Part
ในอาร์เรย์contents
โดยอาจเป็นข้อมูลแบบอินไลน์
(ไฟล์ในเครื่องหรือ URL) และการอ้างอิง File API
Python
from google import genai
from google.genai import types
client = genai.Client()
# Upload the first image
image1_path = "path/to/image1.jpg"
uploaded_file = client.files.upload(file=image1_path)
# Prepare the second image as inline data
image2_path = "path/to/image2.png"
with open(image2_path, 'rb') as f:
img2_bytes = f.read()
# Create the prompt with text and multiple images
response = client.models.generate_content(
model="gemini-2.5-flash",
contents=[
"What is different between these two images?",
uploaded_file, # Use the uploaded file reference
types.Part.from_bytes(
data=img2_bytes,
mime_type='image/png'
)
]
)
print(response.text)
JavaScript
import {
GoogleGenAI,
createUserContent,
createPartFromUri,
} from "@google/genai";
import * as fs from "node:fs";
const ai = new GoogleGenAI({});
async function main() {
// Upload the first image
const image1_path = "path/to/image1.jpg";
const uploadedFile = await ai.files.upload({
file: image1_path,
config: { mimeType: "image/jpeg" },
});
// Prepare the second image as inline data
const image2_path = "path/to/image2.png";
const base64Image2File = fs.readFileSync(image2_path, {
encoding: "base64",
});
// Create the prompt with text and multiple images
const response = await ai.models.generateContent({
model: "gemini-2.5-flash",
contents: createUserContent([
"What is different between these two images?",
createPartFromUri(uploadedFile.uri, uploadedFile.mimeType),
{
inlineData: {
mimeType: "image/png",
data: base64Image2File,
},
},
]),
});
console.log(response.text);
}
await main();
Go
// Upload the first image
image1Path := "path/to/image1.jpg"
uploadedFile, _ := client.Files.UploadFromPath(ctx, image1Path, nil)
// Prepare the second image as inline data
image2Path := "path/to/image2.jpeg"
imgBytes, _ := os.ReadFile(image2Path)
parts := []*genai.Part{
genai.NewPartFromText("What is different between these two images?"),
genai.NewPartFromBytes(imgBytes, "image/jpeg"),
genai.NewPartFromURI(uploadedFile.URI, uploadedFile.MIMEType),
}
contents := []*genai.Content{
genai.NewContentFromParts(parts, genai.RoleUser),
}
result, _ := client.Models.GenerateContent(
ctx,
"gemini-2.5-flash",
contents,
nil,
)
fmt.Println(result.Text())
REST
# Upload the first image
IMAGE1_PATH="path/to/image1.jpg"
MIME1_TYPE=$(file -b --mime-type "${IMAGE1_PATH}")
NUM1_BYTES=$(wc -c < "${IMAGE1_PATH}")
DISPLAY_NAME1=IMAGE1
tmp_header_file1=upload-header1.tmp
curl "https://generativelanguage.googleapis.com/upload/v1beta/files" \
-H "x-goog-api-key: $GEMINI_API_KEY" \
-D upload-header1.tmp \
-H "X-Goog-Upload-Protocol: resumable" \
-H "X-Goog-Upload-Command: start" \
-H "X-Goog-Upload-Header-Content-Length: ${NUM1_BYTES}" \
-H "X-Goog-Upload-Header-Content-Type: ${MIME1_TYPE}" \
-H "Content-Type: application/json" \
-d "{'file': {'display_name': '${DISPLAY_NAME1}'}}" 2> /dev/null
upload_url1=$(grep -i "x-goog-upload-url: " "${tmp_header_file1}" | cut -d" " -f2 | tr -d "\r")
rm "${tmp_header_file1}"
curl "${upload_url1}" \
-H "Content-Length: ${NUM1_BYTES}" \
-H "X-Goog-Upload-Offset: 0" \
-H "X-Goog-Upload-Command: upload, finalize" \
--data-binary "@${IMAGE1_PATH}" 2> /dev/null > file_info1.json
file1_uri=$(jq ".file.uri" file_info1.json)
echo file1_uri=$file1_uri
# Prepare the second image (inline)
IMAGE2_PATH="path/to/image2.png"
MIME2_TYPE=$(file -b --mime-type "${IMAGE2_PATH}")
if [[ "$(base64 --version 2>&1)" = *"FreeBSD"* ]]; then
B64FLAGS="--input"
else
B64FLAGS="-w0"
fi
IMAGE2_BASE64=$(base64 $B64FLAGS $IMAGE2_PATH)
# Now generate content using both images
curl "https://generativelanguage.googleapis.com/v1beta/models/gemini-2.5-flash:generateContent" \
-H "x-goog-api-key: $GEMINI_API_KEY" \
-H 'Content-Type: application/json' \
-X POST \
-d '{
"contents": [{
"parts":[
{"text": "What is different between these two images?"},
{"file_data":{"mime_type": "'"${MIME1_TYPE}"'", "file_uri": '$file1_uri'}},
{
"inline_data": {
"mime_type":"'"${MIME2_TYPE}"'",
"data": "'"$IMAGE2_BASE64"'"
}
}
]
}]
}' 2> /dev/null > response.json
cat response.json
echo
jq ".candidates[].content.parts[].text" response.json
การตรวจจับออบเจ็กต์
ตั้งแต่ Gemini 2.0 เป็นต้นไป โมเดลจะได้รับการฝึกเพิ่มเติมเพื่อตรวจจับออบเจ็กต์ในรูปภาพและรับพิกัดกรอบล้อมรอบ พิกัดที่สัมพันธ์กับขนาดรูปภาพจะปรับขนาดเป็น [0, 1000] คุณต้องยกเลิกการปรับขนาดพิกัดเหล่านี้ตาม ขนาดรูปภาพต้นฉบับ
Python
from google import genai
from google.genai import types
from PIL import Image
import json
client = genai.Client()
prompt = "Detect the all of the prominent items in the image. The box_2d should be [ymin, xmin, ymax, xmax] normalized to 0-1000."
image = Image.open("/path/to/image.png")
config = types.GenerateContentConfig(
response_mime_type="application/json"
)
response = client.models.generate_content(model="gemini-2.5-flash",
contents=[image, prompt],
config=config
)
width, height = image.size
bounding_boxes = json.loads(response.text)
converted_bounding_boxes = []
for bounding_box in bounding_boxes:
abs_y1 = int(bounding_box["box_2d"][0]/1000 * height)
abs_x1 = int(bounding_box["box_2d"][1]/1000 * width)
abs_y2 = int(bounding_box["box_2d"][2]/1000 * height)
abs_x2 = int(bounding_box["box_2d"][3]/1000 * width)
converted_bounding_boxes.append([abs_x1, abs_y1, abs_x2, abs_y2])
print("Image size: ", width, height)
print("Bounding boxes:", converted_bounding_boxes)
ดูตัวอย่างเพิ่มเติมได้ที่สมุดบันทึกต่อไปนี้ในสูตรการแก้ปัญหาของ Gemini
การแบ่งกลุ่ม
ตั้งแต่ Gemini 2.5 เป็นต้นไป โมเดลไม่เพียงตรวจจับไอเทมเท่านั้น แต่ยังแบ่งกลุ่มไอเทม และระบุมาสก์รูปร่างของไอเทมด้วย
โมเดลจะคาดการณ์รายการ JSON โดยแต่ละรายการแสดงถึงมาสก์การแบ่งกลุ่ม
แต่ละรายการมีกรอบล้อมรอบ ("box_2d
") ในรูปแบบ [y0, x0, y1, x1]
โดยมี
พิกัดที่ปรับให้เป็นมาตรฐานระหว่าง 0 ถึง 1000 ป้ายกำกับ ("label
") ที่ระบุ
ออบเจ็กต์ และสุดท้ายคือมาสก์การแบ่งกลุ่มภายในกรอบล้อมรอบเป็น png ที่เข้ารหัส base64
ซึ่งเป็นแผนที่ความน่าจะเป็นที่มีค่าระหว่าง 0 ถึง 255
คุณต้องปรับขนาดมาสก์ให้ตรงกับขนาดกล่องขอบเขต จากนั้น
แปลงเป็นไบนารีที่เกณฑ์ความเชื่อมั่น (127 สำหรับจุดกึ่งกลาง)
Python
from google import genai
from google.genai import types
from PIL import Image, ImageDraw
import io
import base64
import json
import numpy as np
import os
client = genai.Client()
def parse_json(json_output: str):
# Parsing out the markdown fencing
lines = json_output.splitlines()
for i, line in enumerate(lines):
if line == "```json":
json_output = "\n".join(lines[i+1:]) # Remove everything before "```json"
output = json_output.split("```")[0] # Remove everything after the closing "```"
break # Exit the loop once "```json" is found
return json_output
def extract_segmentation_masks(image_path: str, output_dir: str = "segmentation_outputs"):
# Load and resize image
im = Image.open(image_path)
im.thumbnail([1024, 1024], Image.Resampling.LANCZOS)
prompt = """
Give the segmentation masks for the wooden and glass items.
Output a JSON list of segmentation masks where each entry contains the 2D
bounding box in the key "box_2d", the segmentation mask in key "mask", and
the text label in the key "label". Use descriptive labels.
"""
config = types.GenerateContentConfig(
thinking_config=types.ThinkingConfig(thinking_budget=0) # set thinking_budget to 0 for better results in object detection
)
response = client.models.generate_content(
model="gemini-2.5-flash",
contents=[prompt, im], # Pillow images can be directly passed as inputs (which will be converted by the SDK)
config=config
)
# Parse JSON response
items = json.loads(parse_json(response.text))
# Create output directory
os.makedirs(output_dir, exist_ok=True)
# Process each mask
for i, item in enumerate(items):
# Get bounding box coordinates
box = item["box_2d"]
y0 = int(box[0] / 1000 * im.size[1])
x0 = int(box[1] / 1000 * im.size[0])
y1 = int(box[2] / 1000 * im.size[1])
x1 = int(box[3] / 1000 * im.size[0])
# Skip invalid boxes
if y0 >= y1 or x0 >= x1:
continue
# Process mask
png_str = item["mask"]
if not png_str.startswith("data:image/png;base64,"):
continue
# Remove prefix
png_str = png_str.removeprefix("data:image/png;base64,")
mask_data = base64.b64decode(png_str)
mask = Image.open(io.BytesIO(mask_data))
# Resize mask to match bounding box
mask = mask.resize((x1 - x0, y1 - y0), Image.Resampling.BILINEAR)
# Convert mask to numpy array for processing
mask_array = np.array(mask)
# Create overlay for this mask
overlay = Image.new('RGBA', im.size, (0, 0, 0, 0))
overlay_draw = ImageDraw.Draw(overlay)
# Create overlay for the mask
color = (255, 255, 255, 200)
for y in range(y0, y1):
for x in range(x0, x1):
if mask_array[y - y0, x - x0] > 128: # Threshold for mask
overlay_draw.point((x, y), fill=color)
# Save individual mask and its overlay
mask_filename = f"{item['label']}_{i}_mask.png"
overlay_filename = f"{item['label']}_{i}_overlay.png"
mask.save(os.path.join(output_dir, mask_filename))
# Create and save overlay
composite = Image.alpha_composite(im.convert('RGBA'), overlay)
composite.save(os.path.join(output_dir, overlay_filename))
print(f"Saved mask and overlay for {item['label']} to {output_dir}")
# Example usage
if __name__ == "__main__":
extract_segmentation_masks("path/to/image.png")
ดูตัวอย่างโดยละเอียดเพิ่มเติมได้ใน ตัวอย่างการแบ่งกลุ่ม ในคู่มือ Cookbook
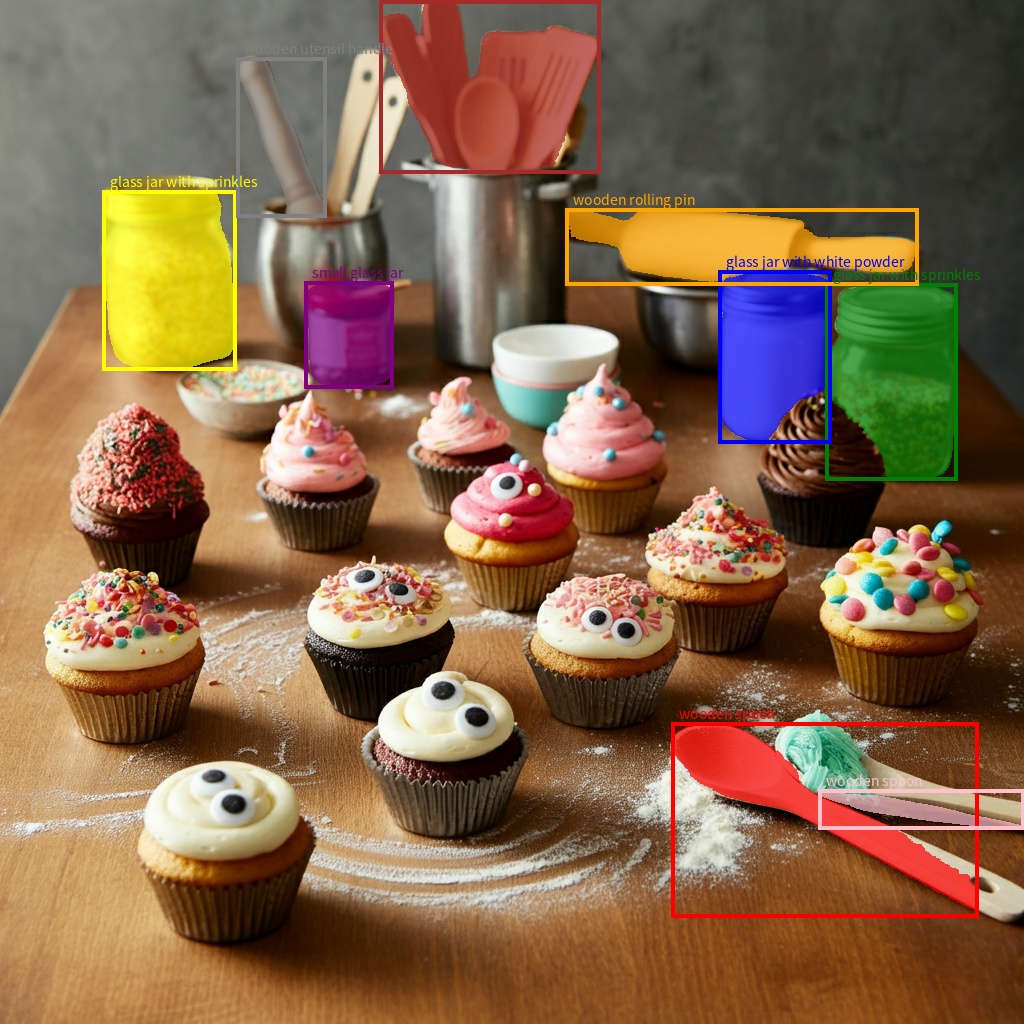
รูปแบบรูปภาพที่รองรับ
Gemini รองรับประเภท MIME ของรูปแบบรูปภาพต่อไปนี้
- PNG -
image/png
- JPEG -
image/jpeg
- WEBP -
image/webp
- HEIC -
image/heic
- HEIF -
image/heif
ความสามารถ
โมเดล Gemini ทุกเวอร์ชันทำงานได้กับข้อมูลหลายรูปแบบและสามารถนำไปใช้ในงานประมวลผลรูปภาพและคอมพิวเตอร์วิทัศน์ได้หลากหลาย ซึ่งรวมถึงแต่ไม่จำกัดเพียงการใส่คำบรรยายแทนรูปภาพ คำถามและคำตอบเกี่ยวกับภาพ การจัดประเภทรูปภาพ การตรวจจับและการแบ่งส่วนออบเจ็กต์
Gemini สามารถลดความจำเป็นในการใช้โมเดล ML เฉพาะทางได้ ทั้งนี้ขึ้นอยู่กับข้อกำหนดด้านคุณภาพและประสิทธิภาพ
โมเดลเวอร์ชันต่อๆ มาบางรุ่นได้รับการฝึกมาโดยเฉพาะเพื่อปรับปรุงความแม่นยำของงานเฉพาะทางนอกเหนือจากความสามารถทั่วไป
โมเดล Gemini 2.0 ได้รับการฝึกเพิ่มเติมเพื่อรองรับการตรวจจับออบเจ็กต์ที่ได้รับการปรับปรุง
โมเดล Gemini 2.5 ได้รับการฝึกเพิ่มเติมเพื่อรองรับการแบ่งกลุ่มที่ได้รับการปรับปรุง นอกเหนือจากการตรวจจับออบเจ็กต์
ข้อจำกัดและข้อมูลทางเทคนิคที่สำคัญ
ขีดจำกัดไฟล์
Gemini 2.5 Pro/Flash, 2.0 Flash, 1.5 Pro และ 1.5 Flash รองรับไฟล์รูปภาพสูงสุด 3,600 ไฟล์ต่อคำขอ
การคำนวณโทเค็น
- Gemini 1.5 Flash และ Gemini 1.5 Pro: 258 โทเค็นหากทั้ง 2 มิติ <= 384 พิกเซล ระบบจะเรียงต่อกันเป็นรูปภาพขนาดใหญ่ (ไทล์ขั้นต่ำ 256 พิกเซล สูงสุด 768 พิกเซล ปรับขนาด เป็น 768x768) โดยแต่ละไทล์จะมีค่าใช้จ่าย 258 โทเค็น
- Gemini 2.0 Flash และ Gemini 2.5 Flash/Pro: 258 โทเค็นหากทั้ง 2 มิติมีขนาดไม่เกิน 384 พิกเซล รูปภาพขนาดใหญ่จะเรียงต่อกันเป็นไทล์ขนาด 768x768 พิกเซล โดยแต่ละไทล์มีค่าใช้จ่าย 258 โทเค็น
เคล็ดลับและแนวทางปฏิบัติที่ดีที่สุด
- ตรวจสอบว่ารูปภาพหมุนอย่างถูกต้อง
- ใช้รูปภาพที่ชัดเจนและไม่เบลอ
- เมื่อใช้รูปภาพเดียวกับข้อความ ให้วางพรอมต์ข้อความหลังส่วนรูปภาพในอาร์เรย์
contents
ขั้นตอนถัดไป
คู่มือนี้จะแสดงวิธีอัปโหลดไฟล์รูปภาพและสร้างเอาต์พุตข้อความจากอินพุตรูปภาพ ดูข้อมูลเพิ่มเติมได้ที่แหล่งข้อมูลต่อไปนี้
- Files API: ดูข้อมูลเพิ่มเติมเกี่ยวกับการอัปโหลดและจัดการไฟล์เพื่อใช้กับ Gemini
- คำสั่งของระบบ: คำสั่งของระบบช่วยให้คุณกำหนดลักษณะการทำงานของโมเดลตามความต้องการและกรณีการใช้งานเฉพาะของคุณได้
- กลยุทธ์การแจ้งไฟล์: Gemini API รองรับการแจ้งด้วยข้อมูลข้อความ รูปภาพ เสียง และวิดีโอ ซึ่งเรียกอีกอย่างว่าการแจ้งแบบมัลติโมดัล
- คำแนะนำด้านความปลอดภัย: บางครั้งโมเดล Generative AI อาจสร้างเอาต์พุตที่ไม่คาดคิด เช่น เอาต์พุตที่ไม่ถูกต้อง มีอคติ หรือไม่เหมาะสม การประมวลผลภายหลังและการประเมินจากเจ้าหน้าที่เป็นสิ่งจำเป็นเพื่อ จำกัดความเสี่ยงที่จะเกิดอันตรายจากเอาต์พุตดังกล่าว