Model Gemini dirancang untuk bersifat multimodal sejak awal, sehingga dapat membuka berbagai tugas pemrosesan gambar dan computer vision, termasuk, tetapi tidak terbatas pada, pemberian teks gambar, klasifikasi, dan proses menjawab pertanyaan visual tanpa harus melatih model ML khusus.
Meneruskan gambar ke Gemini
Anda dapat memberikan gambar sebagai input ke Gemini menggunakan dua metode:
- Menyerahkan data gambar inline: Ideal untuk file yang lebih kecil (total ukuran permintaan kurang dari 20 MB, termasuk perintah).
- Mengupload gambar menggunakan File API: Direkomendasikan untuk file yang lebih besar atau untuk menggunakan kembali gambar di beberapa permintaan.
Meneruskan data gambar inline
Anda dapat meneruskan data gambar inline dalam
permintaan ke generateContent
. Anda dapat memberikan data gambar sebagai string berenkode Base64
atau dengan membaca file lokal secara langsung (bergantung pada bahasa).
Contoh berikut menunjukkan cara membaca gambar dari file lokal dan meneruskannya
ke generateContent
API untuk diproses.
Python
from google.genai import types
with open('path/to/small-sample.jpg', 'rb') as f:
image_bytes = f.read()
response = client.models.generate_content(
model='gemini-2.5-flash',
contents=[
types.Part.from_bytes(
data=image_bytes,
mime_type='image/jpeg',
),
'Caption this image.'
]
)
print(response.text)
JavaScript
import { GoogleGenAI } from "@google/genai";
import * as fs from "node:fs";
const ai = new GoogleGenAI({});
const base64ImageFile = fs.readFileSync("path/to/small-sample.jpg", {
encoding: "base64",
});
const contents = [
{
inlineData: {
mimeType: "image/jpeg",
data: base64ImageFile,
},
},
{ text: "Caption this image." },
];
const response = await ai.models.generateContent({
model: "gemini-2.5-flash",
contents: contents,
});
console.log(response.text);
Go
bytes, _ := os.ReadFile("path/to/small-sample.jpg")
parts := []*genai.Part{
genai.NewPartFromBytes(bytes, "image/jpeg"),
genai.NewPartFromText("Caption this image."),
}
contents := []*genai.Content{
genai.NewContentFromParts(parts, genai.RoleUser),
}
result, _ := client.Models.GenerateContent(
ctx,
"gemini-2.5-flash",
contents,
nil,
)
fmt.Println(result.Text())
REST
IMG_PATH="/path/to/your/image1.jpg"
if [[ "$(base64 --version 2>&1)" = *"FreeBSD"* ]]; then
B64FLAGS="--input"
else
B64FLAGS="-w0"
fi
curl "https://generativelanguage.googleapis.com/v1beta/models/gemini-2.5-flash:generateContent" \
-H "x-goog-api-key: $GEMINI_API_KEY" \
-H 'Content-Type: application/json' \
-X POST \
-d '{
"contents": [{
"parts":[
{
"inline_data": {
"mime_type":"image/jpeg",
"data": "'"$(base64 $B64FLAGS $IMG_PATH)"'"
}
},
{"text": "Caption this image."},
]
}]
}' 2> /dev/null
Anda juga dapat mengambil gambar dari URL, mengonversinya menjadi byte, dan meneruskannya ke
generateContent
seperti yang ditunjukkan dalam contoh berikut.
Python
from google import genai
from google.genai import types
import requests
image_path = "https://goo.gle/instrument-img"
image_bytes = requests.get(image_path).content
image = types.Part.from_bytes(
data=image_bytes, mime_type="image/jpeg"
)
client = genai.Client()
response = client.models.generate_content(
model="gemini-2.5-flash",
contents=["What is this image?", image],
)
print(response.text)
JavaScript
import { GoogleGenAI } from "@google/genai";
async function main() {
const ai = new GoogleGenAI({});
const imageUrl = "https://goo.gle/instrument-img";
const response = await fetch(imageUrl);
const imageArrayBuffer = await response.arrayBuffer();
const base64ImageData = Buffer.from(imageArrayBuffer).toString('base64');
const result = await ai.models.generateContent({
model: "gemini-2.5-flash",
contents: [
{
inlineData: {
mimeType: 'image/jpeg',
data: base64ImageData,
},
},
{ text: "Caption this image." }
],
});
console.log(result.text);
}
main();
Go
package main
import (
"context"
"fmt"
"os"
"io"
"net/http"
"google.golang.org/genai"
)
func main() {
ctx := context.Background()
client, err := genai.NewClient(ctx, nil)
if err != nil {
log.Fatal(err)
}
// Download the image.
imageResp, _ := http.Get("https://goo.gle/instrument-img")
imageBytes, _ := io.ReadAll(imageResp.Body)
parts := []*genai.Part{
genai.NewPartFromBytes(imageBytes, "image/jpeg"),
genai.NewPartFromText("Caption this image."),
}
contents := []*genai.Content{
genai.NewContentFromParts(parts, genai.RoleUser),
}
result, _ := client.Models.GenerateContent(
ctx,
"gemini-2.5-flash",
contents,
nil,
)
fmt.Println(result.Text())
}
REST
IMG_URL="https://goo.gle/instrument-img"
MIME_TYPE=$(curl -sIL "$IMG_URL" | grep -i '^content-type:' | awk -F ': ' '{print $2}' | sed 's/\r$//' | head -n 1)
if [[ -z "$MIME_TYPE" || ! "$MIME_TYPE" == image/* ]]; then
MIME_TYPE="image/jpeg"
fi
# Check for macOS
if [[ "$(uname)" == "Darwin" ]]; then
IMAGE_B64=$(curl -sL "$IMG_URL" | base64 -b 0)
elif [[ "$(base64 --version 2>&1)" = *"FreeBSD"* ]]; then
IMAGE_B64=$(curl -sL "$IMG_URL" | base64)
else
IMAGE_B64=$(curl -sL "$IMG_URL" | base64 -w0)
fi
curl "https://generativelanguage.googleapis.com/v1beta/models/gemini-2.5-flash:generateContent" \
-H "x-goog-api-key: $GEMINI_API_KEY" \
-H 'Content-Type: application/json' \
-X POST \
-d '{
"contents": [{
"parts":[
{
"inline_data": {
"mime_type":"'"$MIME_TYPE"'",
"data": "'"$IMAGE_B64"'"
}
},
{"text": "Caption this image."}
]
}]
}' 2> /dev/null
Mengupload gambar menggunakan File API
Untuk file besar atau agar dapat menggunakan file gambar yang sama berulang kali, gunakan
Files API. Kode berikut mengupload file gambar, lalu menggunakan file dalam
panggilan ke generateContent
. Lihat panduan Files API untuk
mengetahui informasi dan contoh selengkapnya.
Python
from google import genai
client = genai.Client()
my_file = client.files.upload(file="path/to/sample.jpg")
response = client.models.generate_content(
model="gemini-2.5-flash",
contents=[my_file, "Caption this image."],
)
print(response.text)
JavaScript
import {
GoogleGenAI,
createUserContent,
createPartFromUri,
} from "@google/genai";
const ai = new GoogleGenAI({});
async function main() {
const myfile = await ai.files.upload({
file: "path/to/sample.jpg",
config: { mimeType: "image/jpeg" },
});
const response = await ai.models.generateContent({
model: "gemini-2.5-flash",
contents: createUserContent([
createPartFromUri(myfile.uri, myfile.mimeType),
"Caption this image.",
]),
});
console.log(response.text);
}
await main();
Go
package main
import (
"context"
"fmt"
"os"
"google.golang.org/genai"
)
func main() {
ctx := context.Background()
client, err := genai.NewClient(ctx, nil)
if err != nil {
log.Fatal(err)
}
uploadedFile, _ := client.Files.UploadFromPath(ctx, "path/to/sample.jpg", nil)
parts := []*genai.Part{
genai.NewPartFromText("Caption this image."),
genai.NewPartFromURI(uploadedFile.URI, uploadedFile.MIMEType),
}
contents := []*genai.Content{
genai.NewContentFromParts(parts, genai.RoleUser),
}
result, _ := client.Models.GenerateContent(
ctx,
"gemini-2.5-flash",
contents,
nil,
)
fmt.Println(result.Text())
}
REST
IMAGE_PATH="path/to/sample.jpg"
MIME_TYPE=$(file -b --mime-type "${IMAGE_PATH}")
NUM_BYTES=$(wc -c < "${IMAGE_PATH}")
DISPLAY_NAME=IMAGE
tmp_header_file=upload-header.tmp
# Initial resumable request defining metadata.
# The upload url is in the response headers dump them to a file.
curl "https://generativelanguage.googleapis.com/upload/v1beta/files" \
-H "x-goog-api-key: $GEMINI_API_KEY" \
-D upload-header.tmp \
-H "X-Goog-Upload-Protocol: resumable" \
-H "X-Goog-Upload-Command: start" \
-H "X-Goog-Upload-Header-Content-Length: ${NUM_BYTES}" \
-H "X-Goog-Upload-Header-Content-Type: ${MIME_TYPE}" \
-H "Content-Type: application/json" \
-d "{'file': {'display_name': '${DISPLAY_NAME}'}}" 2> /dev/null
upload_url=$(grep -i "x-goog-upload-url: " "${tmp_header_file}" | cut -d" " -f2 | tr -d "\r")
rm "${tmp_header_file}"
# Upload the actual bytes.
curl "${upload_url}" \
-H "x-goog-api-key: $GEMINI_API_KEY" \
-H "Content-Length: ${NUM_BYTES}" \
-H "X-Goog-Upload-Offset: 0" \
-H "X-Goog-Upload-Command: upload, finalize" \
--data-binary "@${IMAGE_PATH}" 2> /dev/null > file_info.json
file_uri=$(jq -r ".file.uri" file_info.json)
echo file_uri=$file_uri
# Now generate content using that file
curl "https://generativelanguage.googleapis.com/v1beta/models/gemini-2.5-flash:generateContent" \
-H "x-goog-api-key: $GEMINI_API_KEY" \
-H 'Content-Type: application/json' \
-X POST \
-d '{
"contents": [{
"parts":[
{"file_data":{"mime_type": "'"${MIME_TYPE}"'", "file_uri": "'"${file_uri}"'"}},
{"text": "Caption this image."}]
}]
}' 2> /dev/null > response.json
cat response.json
echo
jq ".candidates[].content.parts[].text" response.json
Perintah dengan beberapa gambar
Anda dapat memberikan beberapa gambar dalam satu perintah dengan menyertakan beberapa objek
Part
gambar dalam array contents
. Ini dapat berupa campuran data inline
(file lokal atau URL) dan referensi File API.
Python
from google import genai
from google.genai import types
client = genai.Client()
# Upload the first image
image1_path = "path/to/image1.jpg"
uploaded_file = client.files.upload(file=image1_path)
# Prepare the second image as inline data
image2_path = "path/to/image2.png"
with open(image2_path, 'rb') as f:
img2_bytes = f.read()
# Create the prompt with text and multiple images
response = client.models.generate_content(
model="gemini-2.5-flash",
contents=[
"What is different between these two images?",
uploaded_file, # Use the uploaded file reference
types.Part.from_bytes(
data=img2_bytes,
mime_type='image/png'
)
]
)
print(response.text)
JavaScript
import {
GoogleGenAI,
createUserContent,
createPartFromUri,
} from "@google/genai";
import * as fs from "node:fs";
const ai = new GoogleGenAI({});
async function main() {
// Upload the first image
const image1_path = "path/to/image1.jpg";
const uploadedFile = await ai.files.upload({
file: image1_path,
config: { mimeType: "image/jpeg" },
});
// Prepare the second image as inline data
const image2_path = "path/to/image2.png";
const base64Image2File = fs.readFileSync(image2_path, {
encoding: "base64",
});
// Create the prompt with text and multiple images
const response = await ai.models.generateContent({
model: "gemini-2.5-flash",
contents: createUserContent([
"What is different between these two images?",
createPartFromUri(uploadedFile.uri, uploadedFile.mimeType),
{
inlineData: {
mimeType: "image/png",
data: base64Image2File,
},
},
]),
});
console.log(response.text);
}
await main();
Go
// Upload the first image
image1Path := "path/to/image1.jpg"
uploadedFile, _ := client.Files.UploadFromPath(ctx, image1Path, nil)
// Prepare the second image as inline data
image2Path := "path/to/image2.jpeg"
imgBytes, _ := os.ReadFile(image2Path)
parts := []*genai.Part{
genai.NewPartFromText("What is different between these two images?"),
genai.NewPartFromBytes(imgBytes, "image/jpeg"),
genai.NewPartFromURI(uploadedFile.URI, uploadedFile.MIMEType),
}
contents := []*genai.Content{
genai.NewContentFromParts(parts, genai.RoleUser),
}
result, _ := client.Models.GenerateContent(
ctx,
"gemini-2.5-flash",
contents,
nil,
)
fmt.Println(result.Text())
REST
# Upload the first image
IMAGE1_PATH="path/to/image1.jpg"
MIME1_TYPE=$(file -b --mime-type "${IMAGE1_PATH}")
NUM1_BYTES=$(wc -c < "${IMAGE1_PATH}")
DISPLAY_NAME1=IMAGE1
tmp_header_file1=upload-header1.tmp
curl "https://generativelanguage.googleapis.com/upload/v1beta/files" \
-H "x-goog-api-key: $GEMINI_API_KEY" \
-D upload-header1.tmp \
-H "X-Goog-Upload-Protocol: resumable" \
-H "X-Goog-Upload-Command: start" \
-H "X-Goog-Upload-Header-Content-Length: ${NUM1_BYTES}" \
-H "X-Goog-Upload-Header-Content-Type: ${MIME1_TYPE}" \
-H "Content-Type: application/json" \
-d "{'file': {'display_name': '${DISPLAY_NAME1}'}}" 2> /dev/null
upload_url1=$(grep -i "x-goog-upload-url: " "${tmp_header_file1}" | cut -d" " -f2 | tr -d "\r")
rm "${tmp_header_file1}"
curl "${upload_url1}" \
-H "Content-Length: ${NUM1_BYTES}" \
-H "X-Goog-Upload-Offset: 0" \
-H "X-Goog-Upload-Command: upload, finalize" \
--data-binary "@${IMAGE1_PATH}" 2> /dev/null > file_info1.json
file1_uri=$(jq ".file.uri" file_info1.json)
echo file1_uri=$file1_uri
# Prepare the second image (inline)
IMAGE2_PATH="path/to/image2.png"
MIME2_TYPE=$(file -b --mime-type "${IMAGE2_PATH}")
if [[ "$(base64 --version 2>&1)" = *"FreeBSD"* ]]; then
B64FLAGS="--input"
else
B64FLAGS="-w0"
fi
IMAGE2_BASE64=$(base64 $B64FLAGS $IMAGE2_PATH)
# Now generate content using both images
curl "https://generativelanguage.googleapis.com/v1beta/models/gemini-2.5-flash:generateContent" \
-H "x-goog-api-key: $GEMINI_API_KEY" \
-H 'Content-Type: application/json' \
-X POST \
-d '{
"contents": [{
"parts":[
{"text": "What is different between these two images?"},
{"file_data":{"mime_type": "'"${MIME1_TYPE}"'", "file_uri": '$file1_uri'}},
{
"inline_data": {
"mime_type":"'"${MIME2_TYPE}"'",
"data": "'"$IMAGE2_BASE64"'"
}
}
]
}]
}' 2> /dev/null > response.json
cat response.json
echo
jq ".candidates[].content.parts[].text" response.json
Deteksi objek
Mulai Gemini 2.0 dan seterusnya, model dilatih lebih lanjut untuk mendeteksi objek dalam gambar dan mendapatkan koordinat kotak pembatasnya. Koordinat, relatif terhadap dimensi gambar, diskalakan ke [0, 1000]. Anda perlu menskalakan ulang koordinat ini berdasarkan ukuran gambar asli.
Python
from google import genai
from google.genai import types
from PIL import Image
import json
client = genai.Client()
prompt = "Detect the all of the prominent items in the image. The box_2d should be [ymin, xmin, ymax, xmax] normalized to 0-1000."
image = Image.open("/path/to/image.png")
config = types.GenerateContentConfig(
response_mime_type="application/json"
)
response = client.models.generate_content(model="gemini-2.5-flash",
contents=[image, prompt],
config=config
)
width, height = image.size
bounding_boxes = json.loads(response.text)
converted_bounding_boxes = []
for bounding_box in bounding_boxes:
abs_y1 = int(bounding_box["box_2d"][0]/1000 * height)
abs_x1 = int(bounding_box["box_2d"][1]/1000 * width)
abs_y2 = int(bounding_box["box_2d"][2]/1000 * height)
abs_x2 = int(bounding_box["box_2d"][3]/1000 * width)
converted_bounding_boxes.append([abs_x1, abs_y1, abs_x2, abs_y2])
print("Image size: ", width, height)
print("Bounding boxes:", converted_bounding_boxes)
Untuk contoh lainnya, lihat cookbook berikut:
Segmentasi
Mulai Gemini 2.5, model tidak hanya mendeteksi item, tetapi juga menyegmentasikannya dan memberikan mask kontur.
Model memprediksi daftar JSON, dengan setiap item mewakili mask segmentasi.
Setiap item memiliki kotak pembatas ("box_2d
") dalam format [y0, x0, y1, x1]
dengan
koordinat yang dinormalisasi antara 0 dan 1000, label ("label
") yang mengidentifikasi
objek, dan terakhir, mask segmentasi di dalam kotak pembatas, sebagai png yang dienkode base64
yang merupakan peta probabilitas dengan nilai antara 0 dan 255.
Topeng perlu diubah ukurannya agar cocok dengan dimensi kotak pembatas, lalu
di-binerkan pada nilai minimum keyakinan Anda (127 untuk titik tengah).
Python
from google import genai
from google.genai import types
from PIL import Image, ImageDraw
import io
import base64
import json
import numpy as np
import os
client = genai.Client()
def parse_json(json_output: str):
# Parsing out the markdown fencing
lines = json_output.splitlines()
for i, line in enumerate(lines):
if line == "```json":
json_output = "\n".join(lines[i+1:]) # Remove everything before "```json"
json_output = json_output.split("```")[0] # Remove everything after the closing "```"
break # Exit the loop once "```json" is found
return json_output
def extract_segmentation_masks(image_path: str, output_dir: str = "segmentation_outputs"):
# Load and resize image
im = Image.open(image_path)
im.thumbnail([1024, 1024], Image.Resampling.LANCZOS)
prompt = """
Give the segmentation masks for the wooden and glass items.
Output a JSON list of segmentation masks where each entry contains the 2D
bounding box in the key "box_2d", the segmentation mask in key "mask", and
the text label in the key "label". Use descriptive labels.
"""
config = types.GenerateContentConfig(
thinking_config=types.ThinkingConfig(thinking_budget=0) # set thinking_budget to 0 for better results in object detection
)
response = client.models.generate_content(
model="gemini-2.5-flash",
contents=[prompt, im], # Pillow images can be directly passed as inputs (which will be converted by the SDK)
config=config
)
# Parse JSON response
items = json.loads(parse_json(response.text))
# Create output directory
os.makedirs(output_dir, exist_ok=True)
# Process each mask
for i, item in enumerate(items):
# Get bounding box coordinates
box = item["box_2d"]
y0 = int(box[0] / 1000 * im.size[1])
x0 = int(box[1] / 1000 * im.size[0])
y1 = int(box[2] / 1000 * im.size[1])
x1 = int(box[3] / 1000 * im.size[0])
# Skip invalid boxes
if y0 >= y1 or x0 >= x1:
continue
# Process mask
png_str = item["mask"]
if not png_str.startswith("data:image/png;base64,"):
continue
# Remove prefix
png_str = png_str.removeprefix("data:image/png;base64,")
mask_data = base64.b64decode(png_str)
mask = Image.open(io.BytesIO(mask_data))
# Resize mask to match bounding box
mask = mask.resize((x1 - x0, y1 - y0), Image.Resampling.BILINEAR)
# Convert mask to numpy array for processing
mask_array = np.array(mask)
# Create overlay for this mask
overlay = Image.new('RGBA', im.size, (0, 0, 0, 0))
overlay_draw = ImageDraw.Draw(overlay)
# Create overlay for the mask
color = (255, 255, 255, 200)
for y in range(y0, y1):
for x in range(x0, x1):
if mask_array[y - y0, x - x0] > 128: # Threshold for mask
overlay_draw.point((x, y), fill=color)
# Save individual mask and its overlay
mask_filename = f"{item['label']}_{i}_mask.png"
overlay_filename = f"{item['label']}_{i}_overlay.png"
mask.save(os.path.join(output_dir, mask_filename))
# Create and save overlay
composite = Image.alpha_composite(im.convert('RGBA'), overlay)
composite.save(os.path.join(output_dir, overlay_filename))
print(f"Saved mask and overlay for {item['label']} to {output_dir}")
# Example usage
if __name__ == "__main__":
extract_segmentation_masks("path/to/image.png")
Lihat contoh segmentasi dalam panduan cookbook untuk mengetahui contoh yang lebih mendetail.
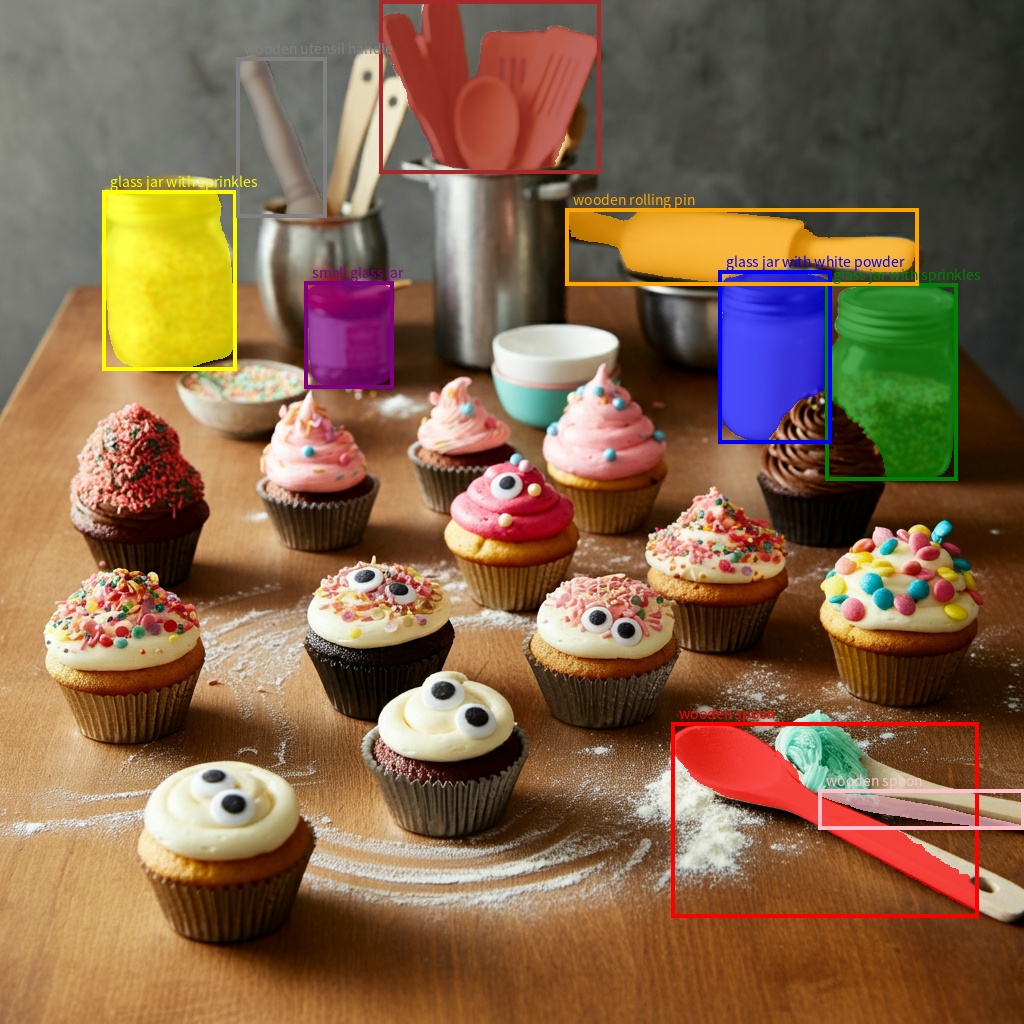
Format gambar yang didukung
Gemini mendukung jenis MIME format gambar berikut:
- PNG -
image/png
- JPEG -
image/jpeg
- WEBP -
image/webp
- HEIC -
image/heic
- HEIF -
image/heif
Kemampuan
Semua versi model Gemini bersifat multimodal dan dapat digunakan dalam berbagai tugas pemrosesan gambar dan computer vision, termasuk, tetapi tidak terbatas pada pemberian teks gambar, pertanyaan dan jawaban visual, klasifikasi gambar, deteksi objek, dan segmentasi.
Gemini dapat mengurangi kebutuhan untuk menggunakan model ML khusus, bergantung pada persyaratan kualitas dan performa Anda.
Beberapa versi model yang lebih baru dilatih secara khusus untuk meningkatkan akurasi tugas khusus selain kemampuan umum:
Model Gemini 2.0 dilatih lebih lanjut untuk mendukung deteksi objek yang ditingkatkan.
Model Gemini 2.5 dilatih lebih lanjut untuk mendukung segmentasi yang ditingkatkan selain deteksi objek.
Batasan dan informasi teknis utama
Batas file
Gemini 2.5 Pro/Flash, 2.0 Flash, 1.5 Pro, dan 1.5 Flash mendukung maksimum 3.600 file gambar per permintaan.
Penghitungan token
- Gemini 1.5 Flash dan Gemini 1.5 Pro: 258 token jika kedua dimensinya <= 384 piksel. Gambar yang lebih besar akan disusun bertingkat (ubin minimum 256 piksel, maksimum 768 piksel, diubah ukurannya menjadi 768x768), dengan setiap ubin berharga 258 token.
- Gemini 2.0 Flash dan Gemini 2.5 Flash/Pro: 258 token jika kedua dimensinya <= 384 piksel. Gambar yang lebih besar akan dipecah menjadi ubin berukuran 768x768 piksel, dengan biaya masing-masing 258 token.
Tips dan praktik terbaik
- Pastikan gambar diputar dengan benar.
- Gunakan gambar yang jelas dan tidak buram.
- Saat menggunakan satu gambar dengan teks, tempatkan perintah teks setelah bagian gambar dalam array
contents
.
Langkah berikutnya
Panduan ini menunjukkan cara mengupload file gambar dan membuat output teks dari input gambar. Untuk mempelajari lebih lanjut, lihat referensi berikut:
- Files API: Pelajari lebih lanjut cara mengupload dan mengelola file untuk digunakan dengan Gemini.
- Petunjuk sistem: Petunjuk sistem memungkinkan Anda mengarahkan perilaku model berdasarkan kebutuhan dan kasus penggunaan tertentu.
- Strategi perintah file: Gemini API mendukung perintah dengan data teks, gambar, audio, dan video, yang juga dikenal sebagai perintah multimodal.
- Panduan keamanan: Terkadang model AI generatif menghasilkan output yang tidak terduga, seperti output yang tidak akurat, bias, atau menyinggung. Pascapemrosesan dan evaluasi manusia sangat penting untuk membatasi risiko bahaya dari output tersebut.